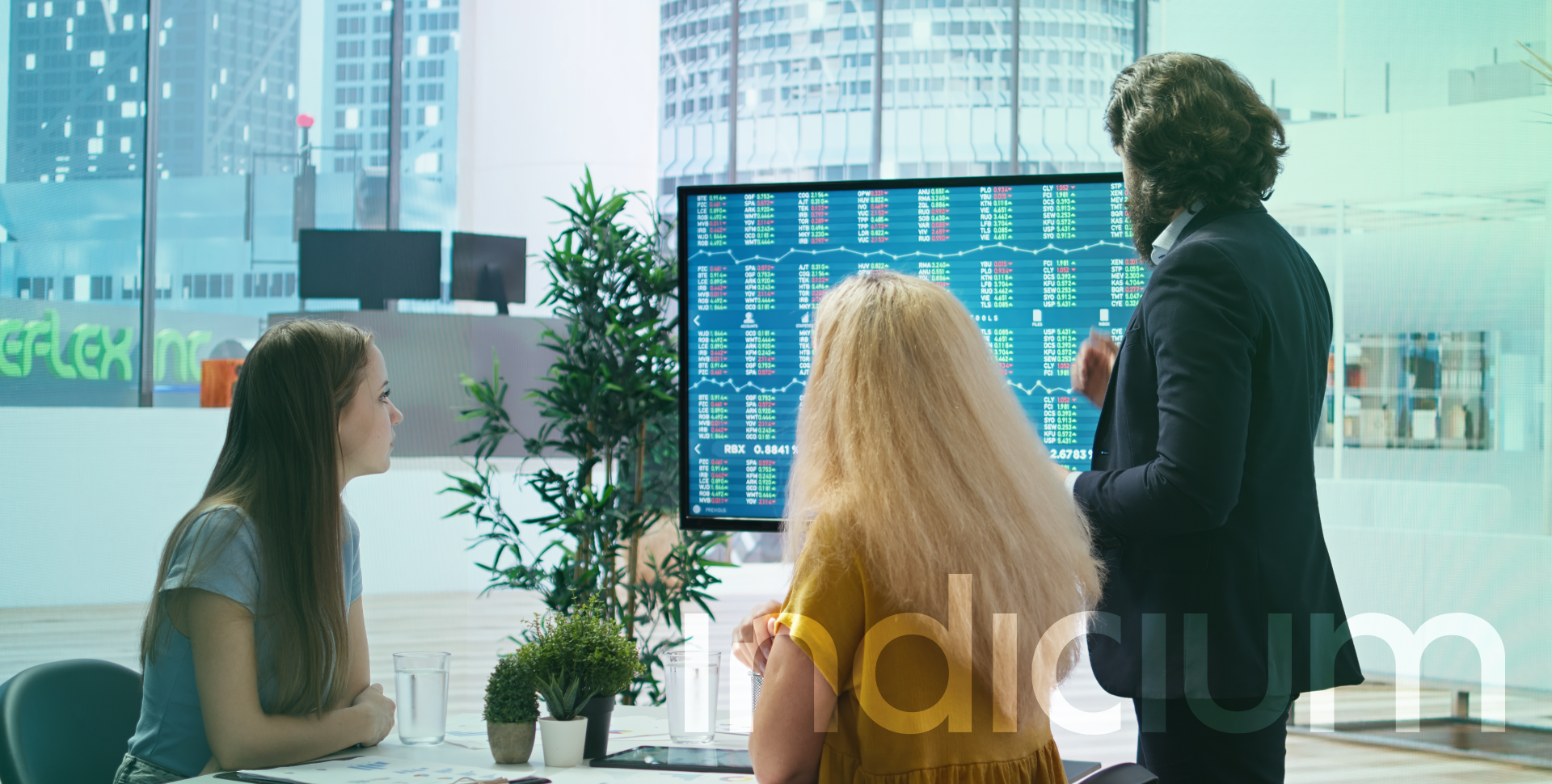
7 Reasons Data Science Projects Fail and How to Fix Them
-
Written by
-
CategoryAI Enablement & Readiness
-
Published DateFebruary 21, 2025
Launching data science projects is one thing. Seeing them through successfully to completion can be quite another. Why? Because a variety of problems – some of which are technical in nature, and others of which stem from collaboration challenges – can cause even the best-planned data science initiatives to go awry.
Data science success hinges, in part, on anticipating these challenges and planning around them. To that end, here’s a look at seven common sources of data science project failure, along with tips on how to avoid letting these pitfalls hamper your next project.
1. Low-Quality Data
Data quality problems – such as data that is incomplete, inconsistent or redundant – are among the most widely known challenges to successful data science projects. But I bring them up nonetheless because there is no overstating how critical it is to ensure data quality as the first step in undertaking a project that hinges on the ability to process, analyze and transform data.
It’s worth noting, too, that just because data is of low quality at the start of a project doesn’t mean the project is bound to fail. There are many effective techniques for improving data quality, such as data cleansing and standardization. When projects fail, it’s typically because they failed to assess data quality and improve it as needed, not because the data was so poor in quality that there was no saving it.
2. Not Knowing Where Data Resides
Another common data science challenge is not knowing exactly where your data exists. Large organizations may own hundreds of data assets spread across sprawling, multi-faceted IT infrastructures. Unless they have a detailed, continuously updated data catalog in place that tracks all of those assets – which many don’t – simply finding the data that the team needs to complete a project can present a major challenge.
Here again, however, tools and techniques are available that can help. The major solution is data discovery software, which can automatically identify data resources, including those that are not documented.
3. Hard-to-Access Data
Sometimes, you know where your data is, but you struggle to access it. This could be because the data resides in a legacy system that is poorly documented or no longer actively supported. Or, the data may be formatted in a way that makes it difficult to read or process.
These are problems that you can work through, but only if you anticipate these challenges from the start of your data science project and deploy the resources necessary to address them. For example, you may need to locate experts who understand legacy systems and can unlock the data stored in them.
4. Lack of Clear Project Goals
So far, I’ve described technical challenges to data science project success. Let’s pivot now to what you might call organizational or behavioral challenges, starting with a common pitfall: A lack of clear project goals.
Too often, businesses decide that they want to do something with their data, but they don’t know exactly what. For example, they might establish a high-level goal like using data-derived insights to grow revenue, without determining exactly which types of revenue-related challenges they want to solve with help from data.
Avoiding this pitfall is simple: You need to articulate precise deliverables and outcomes at the start of your project. There’s always room to adjust the details a bit once a project is underway, but you should know from the beginning what the overarching outcomes of the project should be.
5. Lack of Collaboration Between the IT Department and the Business
There are two key stakeholders in any data science project – the IT department, which is responsible for managing data assets, and business users, who determine what the data science project should achieve.
Unfortunately, poor collaboration between these groups can cause projects to fail. For example, IT departments might decide to impose access restrictions on data without consulting business users, leading to situations where the business can’t actually use the data in the way it intends. Or, lack of input from business stakeholders about what they want to do may cause the IT team to struggle to determine how to deliver the data resources necessary to support a project.
6. Inflexible Project Roadmaps
In a data science project of any scale or complexity, problems are bound to arise, no matter how carefully you plan ahead. Your team may run into issues like unanticipated data quality problems, for example, or find that it’s missing important types of data.
Solving these challenges requires deviating from the original plans. This is not to say that the team needs to rethink its goals and methods altogether, but that it needs to be flexible enough to accommodate change. Otherwise, carefully laid plans become the worst enemy of a successful data science project.
7. Misunderstanding the Goals of Data Science
A final key challenge that can thwart data science project success is the failure to understand what the goals of data science are, and which methodologies and resources data science requires.
For instance, a business might decide that it wants to adopt AI technology. Data science can be a way to achieve this goal if the organization decides to train or customize its own model, for example – and if it invests in the data management infrastructure and tools necessary to support the process.
But if the goal is instead to adopt a third-party AI application or service, data science isn’t necessary. It’s a misuse of the term data science to imply that everything that has to do with data in any way is data science.
To put this another way: Your data science project will only succeed if it’s truly a data science project. If it’s not – if you’re pursuing goals that don’t actually require data science – you may end up investing in data science tools, resources and processes that will never bear fruit, simply because they’re not the solution to your goal.
How to Guarantee Success in Your Data Science Projects
To be sure, there is no “one dumb trick” or simple means of ensuring that your data science project will succeed. But steps like careful management of data quality and data access, setting clear goals and adopting a flexible project framework go far to maximize your odds of success.
This article originally appeared at TechNative under the title ‘Seven Common Reasons Why Data Science Projects Fail’.
About Indicium
Indicium is a global leader in data and AI services, built to help enterprises solve what matters now and prepare for what comes next. Backed by a 40 million dollar investment and a team of more than 400 certified professionals, we deliver end-to-end solutions across the full data lifecycle. Our proprietary AI-enabled, IndiMesh framework powers every engagement with collective intelligence, proven expertise, and rigorous quality control. Industry leaders like PepsiCo and Bayer trust Indicium to turn complex data challenges into lasting results.
Gabriel Klock is Indicium’s Project Management Coordinator. He specializes in data project management, Lean Six Sigma methodologies, and agile frameworks to lead his team in transforming business challenges into successful, impactful projects.
Tags: